How AI Can Help Power Utilities Meet Modern Challenges
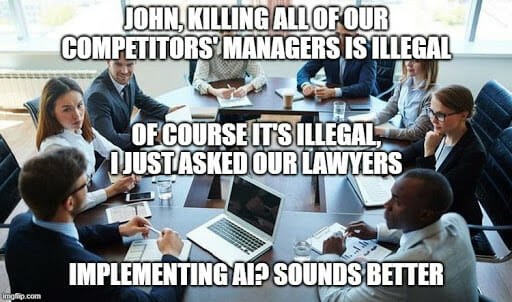
In recent years, electric utilities have faced numerous issues. Above all, increasingly complex and less regulated markets, energy diversification, and growing competition.
How to deal with these challenges? Well, the best solution (without the involvement of hitmen) is probably artificial intelligence.
Specifically, AI can help utilities by:
- Forecasting the energy load
- Optimizing power generation efficiency
- Improving transmission and distribution processes
- Supporting demand management
- Enhancing maintenance tasks
- Transforming the customer experience
Let’s find out how!
Artificial intelligence and the energy revolution
The energy industry as a whole is going through a period of rapid and inexorable change.
Its traditional models based on strict regulation, guaranteed returns, and predictable trends are giving way to a much more “wild” environment.
The energy sector of tomorrow is conceived as a digitized, decentralized, and diversified ecosystem, characterized by real-time price fluctuations and the coexistence of numerous energy sources.
An evolution of this magnitude can only be faced by electric utilities with adequate tools, primarily new economic models and the most innovative technologies available such as AI.
And so came artificial intelligence!
Searching for solutions to these problems, the major players of the energy market have turned to AI technologies in order to maximize the strengths and reduce the flaws of power generation, distribution, and trading processes.
Basically, energy companies’ managers saw “Matrix”, in which intelligent machines harness humans to produce energy, and decided to do the opposite.
Does it count as revenge?
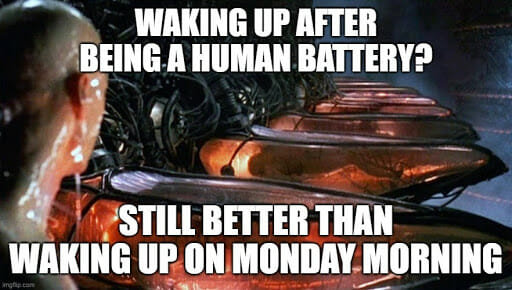
Machine learning and deep learning
The spearheads of these technologies have undoubtedly turned out to be machine learning (ML) and deep learning.
ML is a subpart of AI, specialized in creating computer algorithms that can automatically learn from data and improve their performance through experience.
ML algorithms are able to identify structures and patterns in data sets, build mathematical models regarding these relations, and use them to make predictions or decisions without being previously programmed to do so.
The latest branch of machine learning is deep learning (DP), which mimics the mechanisms of the human brain to process information by using deep artificial neural networks (ANNs).
Why AI… and why now?
Considering the forecasting “superpowers” of AI and ML algorithms, It’s no wonder that these technologies can help utilities cope with the uncertainty and variability of the energy sector.
However, such powers certainly did not come from heaven. The miraculous performances of AI-based systems have been made possible by three fundamental factors.
The first is that massive computational power is now available at low cost and can be used to improve the training speed of deep learning algorithms.
Second, the dramatic increase in available data and storage capacity has provided better training material for algorithms. Ever heard about big data? Exactly, that stuff.
Finally, investments in AI research are growing, particularly in machine learning and deep learning. Cause good intentions are important… but cash is importantER (suffer, English teachers, suffer!).
1. AI to forecast the energy load
After this brief introduction, let’s move on to a general overview of AI’s main fields of application in the energy sector, starting with its forecasting capabilities.
Short-term load forecasts are critical for utilities. Machine learning can be used to predict supply in real-time and optimize distribution.
Nowadays, ML models (SNM, decision trees…) are commonly used to improve forecasting activities and stabilize highly fluctuating production, especially from renewable sources such as solar and wind energy.
Researchers are testing various models under different conditions and locations to identify the most efficient ones, as their performance is still widely debated.
Predicting energy demand with ML
But forecasting can also work on the demand side. This is the case with AutoGrid, which provides insights into energy use based on artificial intelligence.
Its platform is capable of making 10 million forecasts every 10 minutes and optimizing over 50 megawatts of power.
Flex, its core product, processes data from millions of customers with machine learning algorithms to automatically detect supply and demand patterns and suggest adjustments to energy companies.
What does AI-based forecasting imply for trading?
An efficient forecasting system is a pro that should not be underestimated also in financial terms. That’s because programmable power sources are more valuable on the market, as they can provide a certain amount of electricity at a specific time.
This means that accurate forecasts of next day output are essential for the success of financial market operations.
The forecasting and optimization process can also save operators millions of dollars in additional costs or fines for the mismatch between expected and actual production.
In the event of an energy shortage, in fact, grid operators must purchase additional energy on the spot market to supply the grid with prices above the average price … or reimburse governments for their contractual violations.
Remember the last fine you paid for leaving your car in a no-parking area? That was nothing compared to this!
2. AI to optimize power generation
AI isn’t just great at managing Nostradamus-style issues. It can also be used by power providers to improve generation efficiency with automated real-time adjustments.
That’s the case of Siemens’ Gas Turbine Autonomous Control Optimizer, which uses real-time sensor data to monitor conditions in a gas turbine.
This AI-based system performs many different tasks thanks to algorithms, such as controlling the combustion process by learning to constantly adjust fuel valves based on both internal and external variables.
According to tests, this approach ensures better performance and lower emissions (up to 20% reduction in nitrogen oxides).
3. AI to improve distribution processes
The abilities of AI and ML algorithms to predict fluctuations in the energy load would not be so useful if we didn’t have the tools to adapt energy distribution to these variations. Fortunately, these tools exist and, once again, are largely based on artificial intelligence!
We’re talking about smart grids, automated power grids that can monitor and control every consumer and node, providing greater control and flexibility. This ensures a no-stop flow of information on the system status.
The process of monitoring is based on a wide network of smart meters assigned to each user, as well as synchrophasors that measure voltage and current in various points of the grid.
These sensors allow the grid to change the flow of electricity as needed, lowering the workload and energy prices too.
According to AMR (Allied Market Research), the smart grid market is set to grow from $ 67 billion in 2017 to $ 170 billion by 2025.
The US Department of Energy alone has already invested over $ 4.5 billion in such technologies!
“The smart grid market is set to grow from $ 67 billion in 2017 to $ 170 billion by 2025.”
What about storage issues?
Algorithms are also reliable tools for dealing with storage problems.
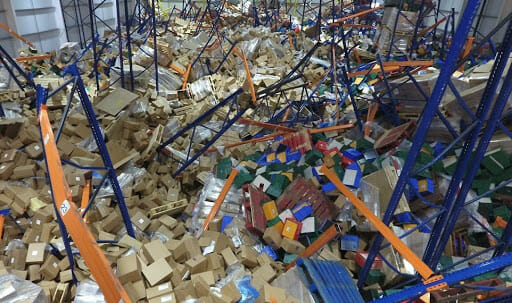
No, not THOSE storage problems. I mean energy storage problems.
Energy storage technology plays a fundamental role especially in the renewable energy sector, which is subject to strong production fluctuations.
Basically, we are talking about intelligent storage units powered by AI and adjustable according to the supply flow.
Artificial intelligence and especially machine learning, by creating models based on previously collected data, can help manage these flows and store excess energy to avoid grid overloads.
4. AI to support demand management
Improving energy production and distribution is certainly cool. But… What if we could reduce energy consumption?
Actually, demand management is already seeing an increasing implementation of AI-powered tools to improve energy management systems and overall energy efficiency.
For example, we can combine energy flow control systems with large industrial equipment, such as air conditioning units and furnaces, to automatically shut them down when power is low.
Another case is that of Google and its AI-focused company DeepMind, which managed to reduce the energy consumption of data centers by 15%.
Google declared it has already saved 40% of the energy consumed for cooling thanks to AI.
5. AI to enhance maintenance tasks
AI has proven to be a talented clairvoyant, an outstanding organizer… and the utilities’ best mechanic!
In fact, AI can help utilities with maintenance processes by replacing time-intensive and risky manual inspections, also via drones and robotics technology.
Recent developments in sensors and signal processing systems, combined with machine learning algorithms, offer new opportunities for efficient and rapid condition monitoring (CM).
CM examines the various activities of power facilities and grids, collecting data by specific sensors and processing it through a wide range of ML-based methodologies to detect malfunctions.
In particular, neural networks (NN), support vector machines (SVM), and decision trees are the most commonly used.
Detecting faults with algorithms
That’s the kind of approach chosen by San Diego Gas & Electric, an important utility that serves 20 million Americans.
This company was facing a massive problem of energy leakage and consequent revenue losses.
Instead of sending technical personnel every five minutes, they decided to implement AI and machine learning to analyze datasets from their grids and detect any possible fault.
Did it work? Well, considering that I’m writing about it, yes, it worked.
The company quickly resolved outage issues, customers were satisfied, the brand value was maintained, and the CEO’s daughter married Prince Charming. Happy ending!
6. AI to transform the customer experience
I know, the list is already quite long, but let me spend a few words on the retail side of the energy market.
Artificial intelligence has the potential to (positively) transform the customer experience and improve user retention.
Utilities can process huge amounts of users’ data thanks to ML algorithms and get insights into their preferences, habits, and discomforts.
This precious information will be used to predict customers’ churn, develop personalized offers, and offer targeted rewards.
AI, call centers, and customer care
AI is also improving the user experience by revolutionizing call centers, which can exploit modern technologies to respond to consumer queries and provide immediate assistance.
“No, sir, that’s a restaurant receipt, not your electricity bill.”
That kind of assistance. For that kind of query.
Another AI-connected advantage for customers is the selection of suppliers. User data can be cross-referenced with that of electricity companies to identify the offers best suited to their needs.
Lumator performs exactly this task by using software developed at Carnegie Mellon University and taking care of the switch without supply interruptions.
What is wrong with AI?
Until now, artificial intelligence looks as cool and perfect as any applicant according to their CV. Yep, it IS cool, but with a couple of flaws.
As already mentioned when talking about smart grids, data is the real fuel of AI-powered systems. But data is a strong point until it is stolen. Data protection and security issues are some of the major weaknesses in the implementation of AI in the energy sector.
For example, in 2018 the German Federal Office for Security recorded an abrupt increase in cyber disruptions on critical infrastructures, including energy facilities, compared to the previous year.
That’s why cybersecurity is becoming increasingly important to protect highly networked power grids from hacker attacks.
On the other hand, AI can also fortify our defenses against cyberattacks, learning from large amounts of data and thus spotting deviations such as the presence of Trojans.
“In 2018 the German Federal Office for Security recorded an abrupt increase in cyber disruptions on critical infrastructures, including energy facilities, compared to the previous year.”
Problems regarding privacy and energy consumption
A second data-related weakness concerns the handling of private user information by energy companies, which seems to be one of the main obstacles to the acceptance of smart meters.
Apparently, for many people, their energy consumption data is even more sensitive than their Google search history. If you know what I mean…
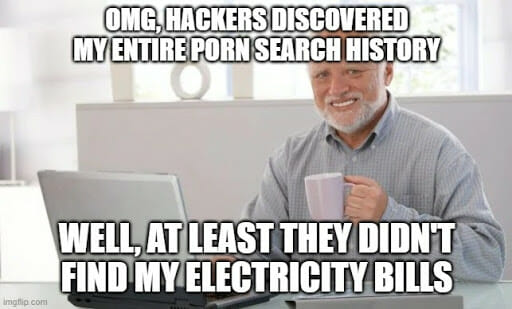
Actually, many state and international institutions have tried to face this challenge with targeted legislative interventions in the field of privacy protection.
The most important milestone, achieved in recent years, is the General Data Protection Regulation (GDPR) in the European Union.
The criticisms are not only focused on the data itself, but also on the power consumption of AI-based systems to handle huge amounts of information. One possible solution is to design data centers to make them energy efficient and possibly powered by renewable energy.
These issues shouldn’t stop AI implementation
The problems described above, combined with the investment risks and the massive costs of AI, seem to partly hold back the rise of these technologies in the energy sector.
According to the research this industry has underestimated the true potential of AI, which could help companies saving between $237 billion to $813 billion from intelligent automation at scale.
For now, the energy and utility industry is struggling to scale their automation initiatives: only 15% have been able to implement multiple use cases at scale.
On the other hand, this sector has made significant progress in adopting AI solutions, rising from just 28% of energy operators in 2017 with few or more AI use cases, to 52% today.
Survival through adaptation
The long-term objective will therefore be to continue this positive trend, cause it’s the only way for utilities to adapt to the new energy market and meet the challenges of tomorrow.
And as Darwin said, it’s not the strongest who survives, but the one who can adapt best.
Will energy companies be like dinosaurs… or like mammals?
Need support with your software development?
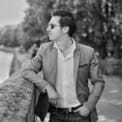
Andrea Di Stefano
Andrea is a journalist and teacher of Italian with a long-standing interest in tech and media. He loves to handle touchscreens, but to correct his students’ homework he prefers the good old red marker on paper!