Why Machine Learning Is Agriculture’s New Best Friend
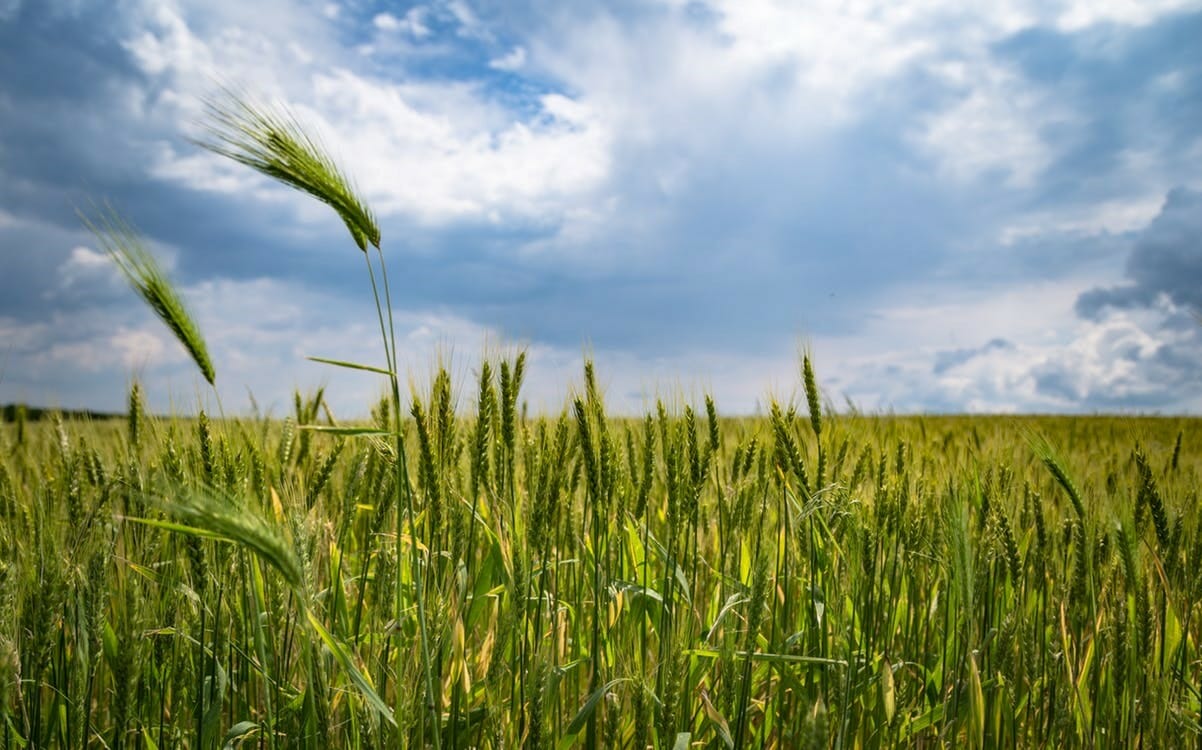
What if I told you that countryside farmers and nerdy programmers can be great colleagues?
You would be surprised to know the incredible contribution that IT is giving to the agricultural sector.
Wanna be surprised?
I know you want it. So, go on!
Data and… tractors
Today, thanks to the application of artificial intelligence and machine learning (ML), agriculture can count on a wider variety of data and analytics tools.
These data are provided by many different types of sensors that allow a better understanding of the environment (crops, weather conditions, soil …) and the agricultural operations (machine data).
By feeding machine learning systems with the collected data, farm management can be supported with real-time recommendations elaborated via artificial intelligence.
Data-intensive approaches allow better decision-making, greater efficiency, and reduced waste while minimizing negative effects for the environment.
Tractor + green paint = John Deere
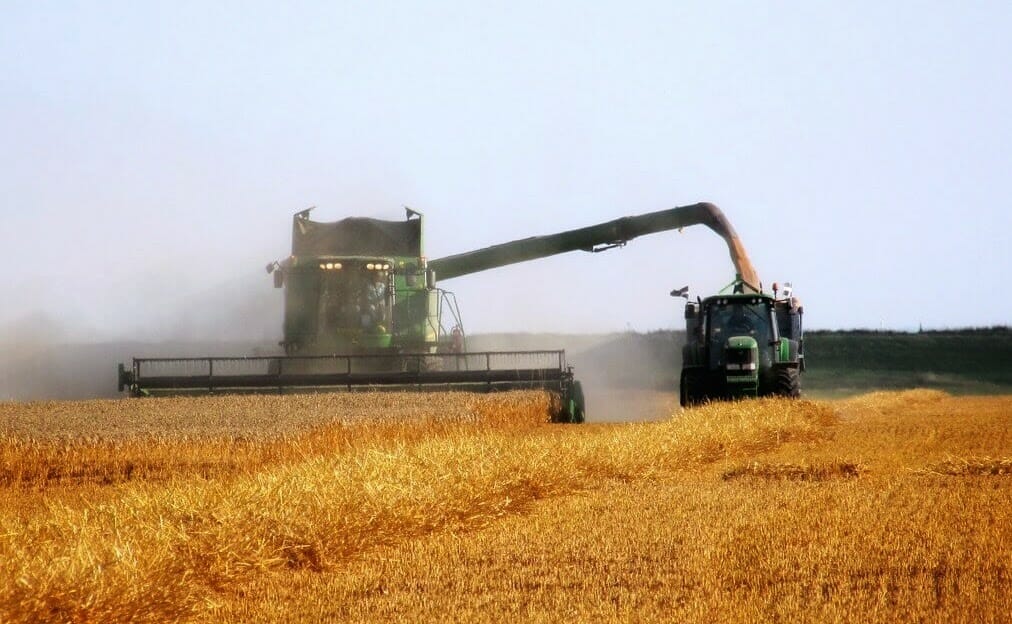
“Data-intensive approaches allow better decision-making, greater efficiency, and reduced waste while minimizing negative effects for the environment.”
What is Digital Agriculture?
This innovative approach, which encompasses machine learning, data science, and artificial intelligence together with a wide range of other technologies, is known as digital agriculture or smart farming.
Yes, if we have smartphones, why can’t we also have smart farming?
If you’ve come this far, I expect you already know the meaning of machine learning and its main types (check the links for more details about this topic).
Let’s say, in a very brief way, that ML is practically a branch of artificial intelligence. It explores the study and construction of algorithms that are able to learn from data and make predictions about them.
It is widely applied in computer programming, providing systems the ability to automatically learn and improve from experience without being explicitly programmed.
ML applications in Agriculture
Ok, it’s time to analyze in detail the practical contribution of machine learning to the agricultural sector. Its applications can be divided into four macro-categories:
- Crop management
- Livestock management
- Water management
- Soil management
The opening scene of Interstellar? Nah, just an agricultural drone.
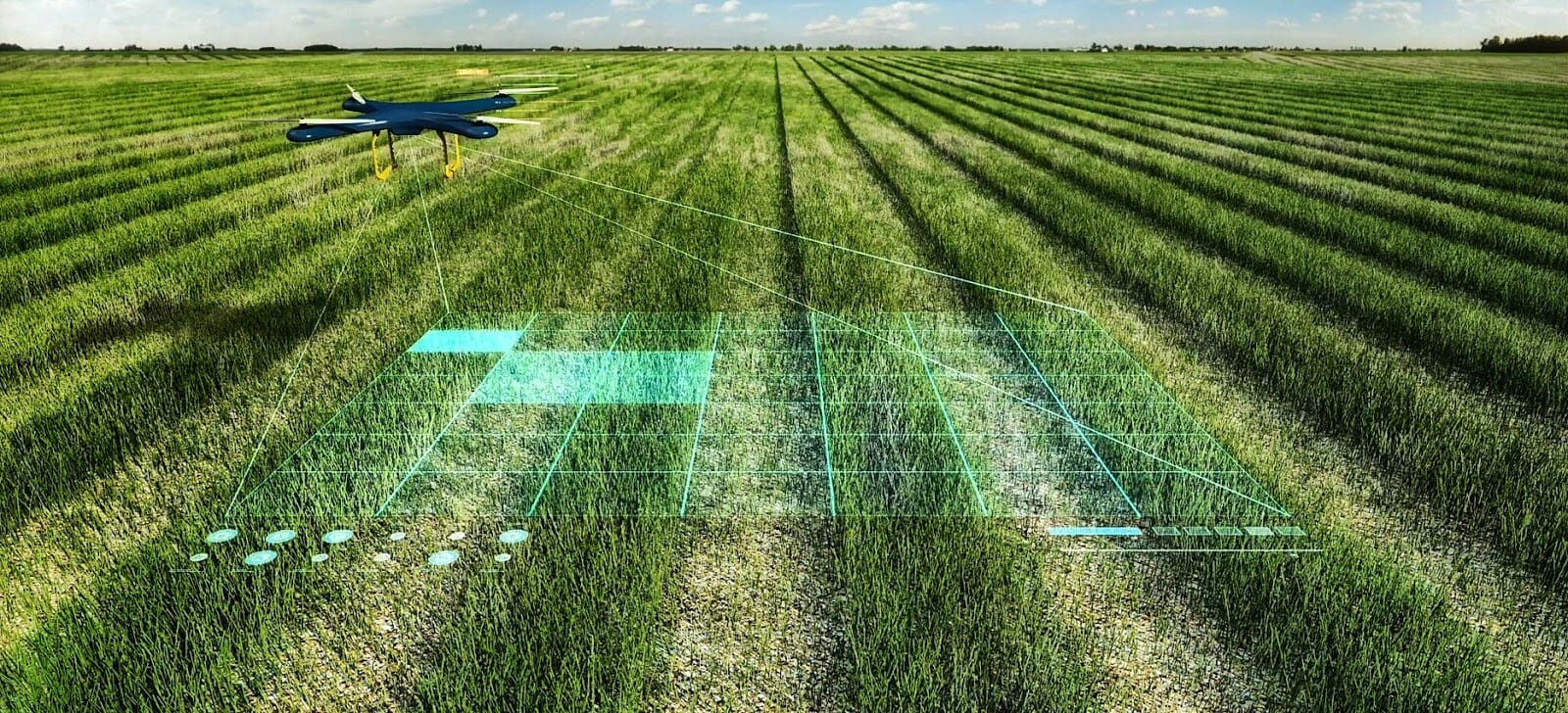
Crop management
Using sensors (mounted on drones, for example) and ML digital applications, farmers can predict harvest yields, make evaluations about the crop quality, detect crop diseases or weed infestations, and identify plant species.
Yield prediction:
It is related to yield mapping and estimation, matching of crop supply with demand, and crop management to increase productivity.
For example, the rice development stage prediction based on SVM (aka “support vector machine”, a type of supervised machine learning model) and basic geographic information obtained from weather stations.
Disease and weed detection:
Thanks to ML-driven image processing, it’s possible to recognize weed species (not THAT weed, shame on you!) and to determine which crops are infested by fungi, bacteria, or viruses.
Furthermore, digital applications can provide an accurate disease diagnosis and recommend the best treatments.
For example, the approach based on a SOM neural network and hyperspectral reflectance imaging to discriminate between the plant stress caused by disease and nutrient deficiency stress.
Species identification:
The automatic identification and classification of plant species is an effective way to avoid the use of human experts, reducing the classification time.
Crop quality:
The accurate detection and classification of crop quality
characteristics can increase product price and reduce waste
A method concerning this topic is based on ML techniques applied to chemical components of samples.
It is common for the prediction and classification of the geographical origin of rice.
Livestock management
ML technology improves farming and ranching operations in many different areas, including livestock health maintenance, animal herding, dairy production, and selective breeding.
Animal welfare:
It focuses on monitoring animal behavior for the early detection of diseases.
This kind of ML-based system can trace various activities of the animals with depth video cameras, including standing, moving, feeding, playing Cluedo…
It’s also possible to analyze the cattle behavior using data collected by collar sensors with magnetometers and three-axis accelerometers, to predict events such as dietary changes.
Livestock production:
The ML-driven analysis helps optimize the production system of milk, eggs, meat, and other dairy products through the accurate prediction and estimation of farming parameters.
Researchers developed an interesting solution to identify pigs without using radio frequency identification tags, which have limited range and require a stressful procedure for the animals.
The system is based on convolutional neural networks, applied in digital images for face recognition.
Some cute cows with tags. And a smarter face than mine on Monday morning.
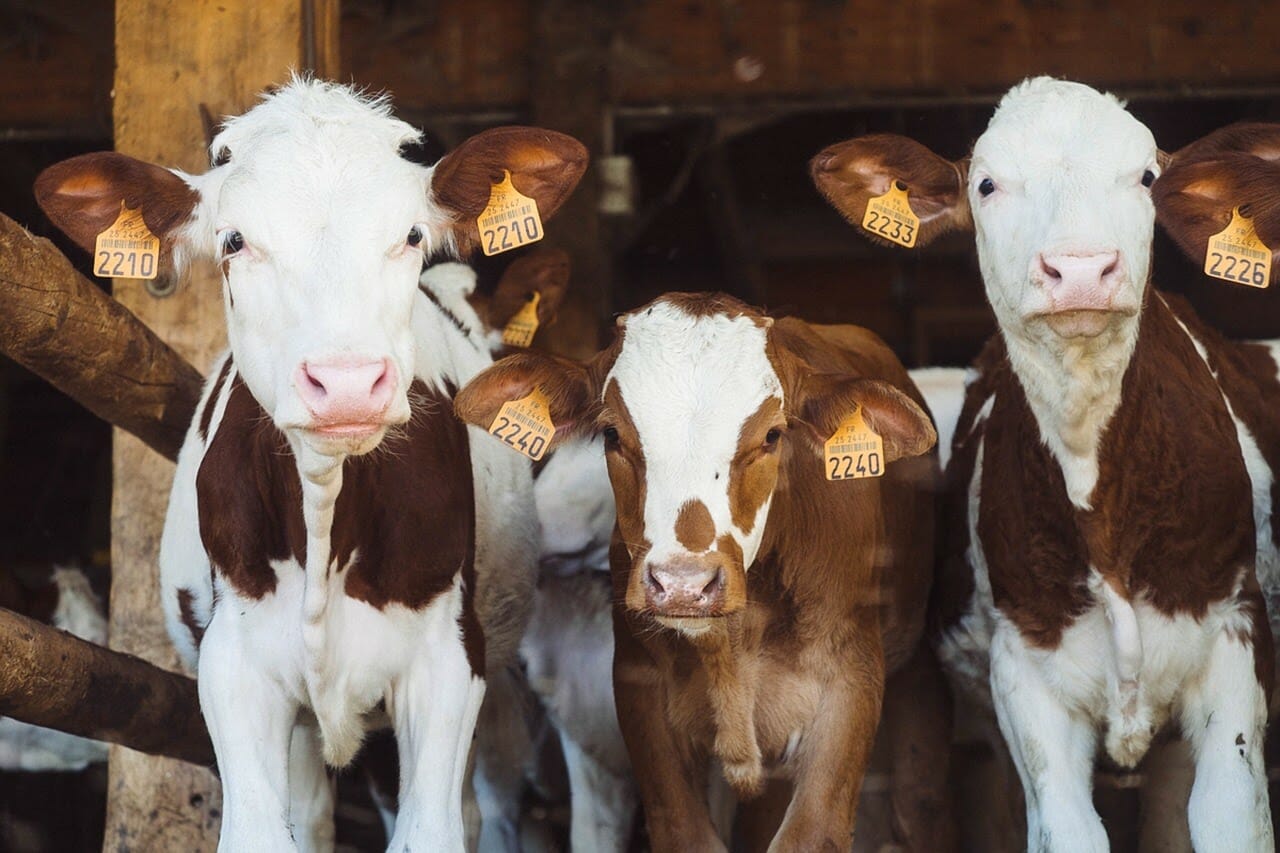
Water management
According to the World Bank, 70% of global freshwater is destined for agriculture. My wife would say that the remaining 30% is for my morning showers.
Feeding an estimated world population of 9-10 billion people in 2050 will require a 50% increase in agricultural production and a 15% increase in water withdrawals.
This can give us an idea of why it is essential to optimize water consumption.
How to save water with machine learning?
Technologists are able to develop efficient irrigation systems thanks to ML-based analysis of precipitations or plant and soil transpiration (known as evapotranspiration, learn this term if you want to impress your friends).
In fact, the correct estimation of evapotranspiration requires complex calculations and is extremely important for the optimization of resource management in crop production.
If implemented in the irrigation control systems, this data allows us to set up strategies that help minimize water usage.
For example, a team of Iranian researchers focused on computational methods to estimate the average monthly evapotranspiration in arid regions.
To do this, they analyzed monthly climate data from 44 weather stations for the period 1951–2010.
Soil management
Soil management can use ML to predict and identify agricultural soil properties like composition, drying, temperature, and moisture.
This approach, based on computational analysis, offers a low cost and less time-consuming solution.
The levels of soil temperature and drying offer important information about climate change effects on a region.
Instead, soil composition and moisture are determinating for crop yield variability.
Machine learning regression models have been used by a team of Greek scientists for the prediction of soil organic carbon, moisture content, and total nitrogen.
Thanks to infrared spectrophotometers, it was possible to collect soil spectra from 140 samples of the top layer of Luvisol soil types (very common in agriculture, even if it sounds more like the name of a drug).
The study demonstrated that the correct prediction of soil properties can optimize soil management. Good job, Greek nerds!
Future challenges for Agriculture
Humanity is gonna face two major challenges in the future.
Challenges that will greatly involve agriculture:
1/
The global food system shall be able to provide food to a population that will grow from the actual 7.5 billion people to nearly 10 billion by 2050.
In addition to plain population growth, we should consider an
increase in demand for meat and fish in emerging economies.
2/
At the same time, it will be difficult to increase the harvest because of global warming, water scarcity, and mass urbanization.
Speaking of global warming, we can count numerous negative effects like the increase of heatwaves, changes in pests and diseases, the sea level rise, and a general worsening in the nutritional quality of some foods.
Not to mention the stink of sweat on the subway.
“The global food system shall be able to provide food to a population that will grow from the actual 7.5 billion people to nearly 10 billion by 2050.”
The entire concept of “forever alone” in one single pic.
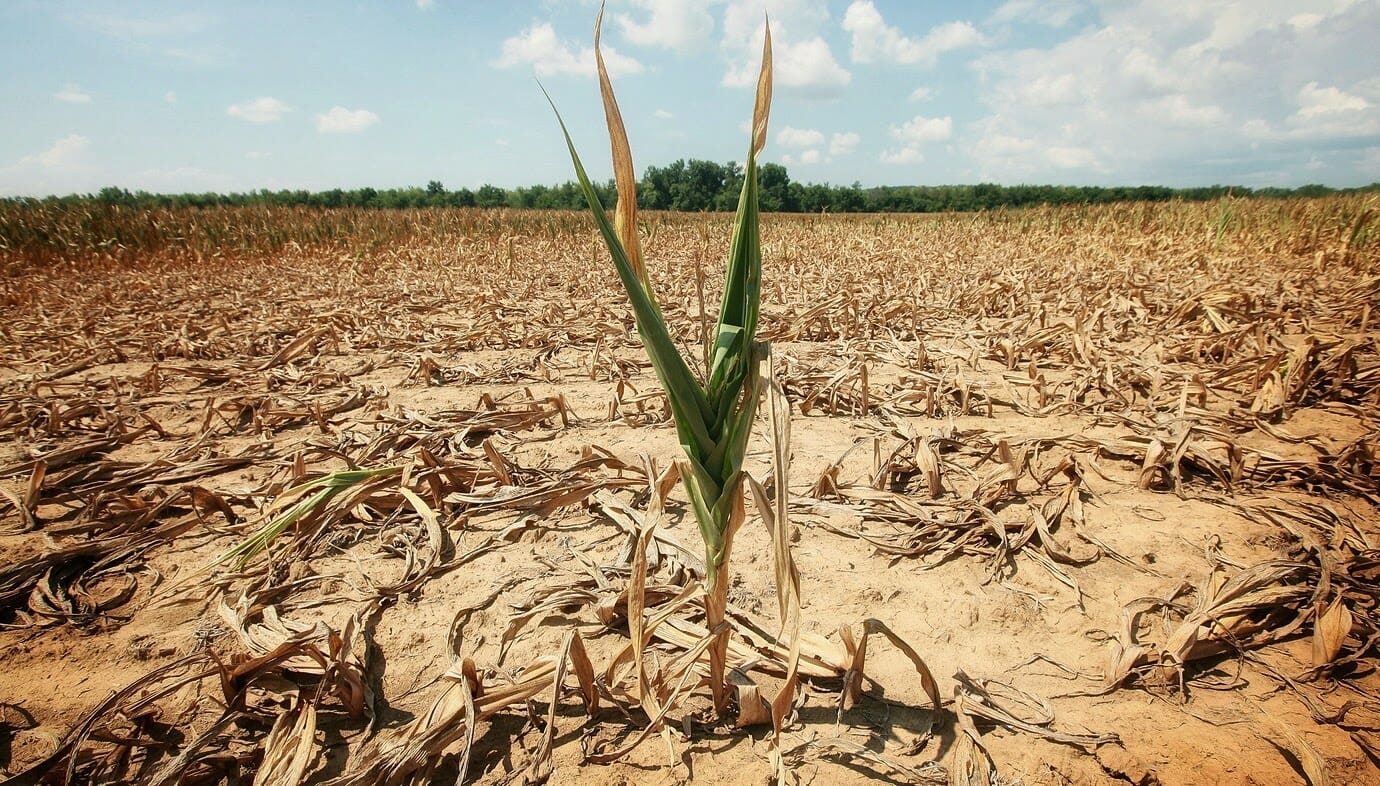
Machine learning is the answer
One of our best hopes to rethink our agricultural systems and be prepared for these challenges is machine learning.
The ability of ML to boost agricultural productivity while minimizing its environmental impact can guarantee humanity the potential to achieve food security.
So yes, digital agriculture will save us.
And yes, farmers and AI developers are great buddies!
Need support with your software development?
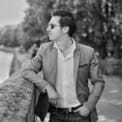
Andrea Di Stefano
Andrea is a journalist and teacher of Italian with a long-standing interest in tech and media. He loves to handle touchscreens, but to correct his students’ homework he prefers the good old red marker on paper!